Overview
- Once candidate generation is complete, the recommendation system uses another model to score and rank the generated candidates in order to select a set of items to present to the user. To achieve this, the system may utilize multiple candidate generators that draw from different sources, such as related items from a matrix factorization model, user-specific features for personalization, geographic information for considering “local” versus “distant” items, popular or trending items, and social graph data that considers items liked or recommended by friends. These various sources are combined into a single pool of candidates, which is then scored and ranked by a single model.
- For instance, the system can train a model that predicts the likelihood of a user watching a video on YouTube based on query features (e.g. user watch history, language, country, time) and video features (e.g. title, tags, video embedding). Let’s look at these different source/inputs used for candidate generation:
- Related items from a matrix factorization model: This approach uses a matrix factorization technique to extract latent factors that can represent user preferences and item attributes. The matrix is factorized into two matrices, one representing users and the other representing items, and their dot product generates a score for each user-item pair. This approach can generate recommendations based on users’ past interactions and can identify items that are similar to the ones that the user has previously interacted with.
- User features that account for personalization: User features such as age, gender, and past search queries can be used to generate personalized recommendations. By analyzing the user’s past interactions and search behavior, a recommendation system can identify items that are likely to be relevant to the user.
- “Local” vs “distant” items; that is, taking geographic information into account: This approach takes into account the user’s geographic location to identify items that are geographically close to the user or are relevant to the user’s location. For example, a recommendation system for a restaurant app can use the user’s location to identify nearby restaurants.
- Popular or trending items: This approach recommends items that are currently popular or trending based on factors such as sales, views, or social media activity. This approach can be useful for introducing users to new and popular items.
- A social graph; that is, items liked or recommended by friends: This approach uses the social connections of the user to identify items that are recommended or liked by the user’s friends or social network. This approach can be particularly useful for social media and e-commerce applications, where users may be influenced by the recommendations of their friends and peers.
- The system takes these multitude of candidates, places them in a common pool of candidates that are scored by a single model and ranked according to that score.
- “For e.g., the system can train a model to predict the probability of a user watching a video on YouTube given the following:
- query features (for example, user watch history, language, country, time)
- video features (for example, title, tags, video embedding)
- The system can then rank the videos in the pool of candidates according to the prediction of the model.” (source)
- Scoring is typically more focused on personalized recommendations and may use more sophisticated machine learning models to capture complex user preferences and item relationships. For example, a scoring model may use a deep neural network to learn complex patterns in user behavior and item features, or it may incorporate contextual information such as time of day or location.
Scoring vs. Ranking
- In recommender systems, scoring and ranking are two key concepts that are used to determine the relevance of items to recommend to a user.
- Scoring refers to the process of assigning a score or a rating to each item in the candidate pool based on its similarity to the user’s preferences or past behavior. The scoring function can be based on different factors such as content similarity, collaborative filtering, or a combination of both. The scoring function is used to determine the relevance of each item to the user, and items with higher scores are considered to be more relevant.
- Ranking, on the other hand, is the process of ordering the items based on their scores. The items with the highest scores are ranked at the top of the recommendation list, while the items with the lowest scores are ranked at the bottom. The ranking process ensures that the most relevant items are presented to the user first.
- To illustrate this, let’s consider an example. Suppose a user is looking for movie recommendations based on their past viewing history. The scoring function may assign a score to each movie in the candidate pool based on factors such as genre, cast, director, and plot. The movies with higher scores would be considered more relevant to the user. The ranking process would then order the movies based on their scores, with the highest-scored movies appearing at the top of the recommendation list.
- In summary, scoring determines the relevance of each item in the candidate pool, while ranking orders the items based on their scores to present the most relevant items to the user.
Why Not Let the Candidate Generator Score?
- “Since candidate generators compute a score (such as the similarity measure in the embedding space), you might be tempted to use them to do ranking as well. However, you should avoid this practice for the following reasons:” (source)
- Using candidate generators for ranking is not a good practice because a recommendation system may have multiple candidate generators that use different sources. The scores generated by these different generators may not be comparable, making it challenging to rank the candidates. Moreover, with a smaller pool of candidates, the system can use more features and a more complex model, which can better capture the context and provide more accurate recommendations. Therefore, it is more appropriate to use a separate model for scoring and ranking the candidates.
Objective Function for Scoring
- Remember, an objective function is a mathematical function used to evaluate how well a machine learning model is performing on a task. It measures the difference between the predicted outputs of the model and the true outputs. The goal of the machine learning algorithm is to minimize or maximize the objective function, depending on whether it is a cost function or a reward function, respectively. The objective function is chosen based on the problem being solved and the specific goals of the model.
- Selecting an objective function for scoring in a recommendation system is a crucial step that requires careful consideration. In machine learning, the objective function is like a genie that learns whatever the user wishes, but the user should be mindful of what they ask for. Similarly, the choice of scoring function in a recommendation system can significantly impact the ranking of items and the overall quality of the recommendations.
- The scoring function should be designed to capture the user’s preferences and produce accurate predictions of the likelihood that a user will interact with a particular item. The objective function can be based on various factors such as user preferences, historical data, or contextual information.
- It is essential to evaluate the performance of different objective functions and select the one that produces the best results in terms of accuracy and relevance. Additionally, the objective function should be flexible enough to handle different types of input data and account for changes in user preferences over time.
- The choice of scoring function in recommendation systems can significantly impact the quality of recommendations.
- For instance, if the scoring function is focused on maximizing click rates, the system may recommend click-bait videos that do not provide a good user experience and can quickly lose users’ interest.
- Similarly, optimizing for watch time alone may lead to recommendations of very long videos, also leading to a poor user experience.
- Alternatively, the system can aim to increase diversity and maximize session watch time by recommending shorter videos that are more likely to keep the user engaged.
- Maximizing click rate might lead to recommending clickbait content that can harm the user experience. Maximizing watch time might lead to recommending long content that might bore the user. A better approach is to balance watch time and diversity, recommending shorter videos that are more likely to keep the user engaged.
Scoring
- Let’s look at different methods and techniques used for scoring.
- Cosine Similarity: A common similarity measure used in content-based filtering to calculate the similarity between the features of items and user preferences.
- Weighted Average Score: Weighted average is a method of calculating a single score for a set of items, where each item is assigned a weight based on its importance and so, this means that items that are more relevant to the user should have a higher weight in the calculation of the final score. To use weighted average in a recommender system, first, the relevance of each item to the user needs to be determined. This can be done through user feedback, such as ratings, reviews, or clicks. Each item is assigned a relevance score based on this feedback.
- Next, weights are assigned to each item based on their importance. The importance can be determined based on different factors, such as popularity, novelty, or profitability. For example, popular items can be assigned a higher weight because they are likely to be more relevant to a larger number of users.
- Finally, the weighted average score is calculated by multiplying the relevance score of each item by its weight, summing up the products, and dividing the result by the sum of weights. The resulting score represents the overall relevance of the set of items to the user.
- Weighted average can be used for both scoring and ranking in a recommender system. In scoring, the weighted average score can be used to represent the relevance of a set of items to a user. In ranking, the items can be sorted based on their weighted average scores, with the highest scoring items appearing at the top of the list.
- Factorization Machines: A popular algorithm for scoring that models interactions between features and allows for non-linear relationships.
- The table below does a comparative analysis between these different methods.
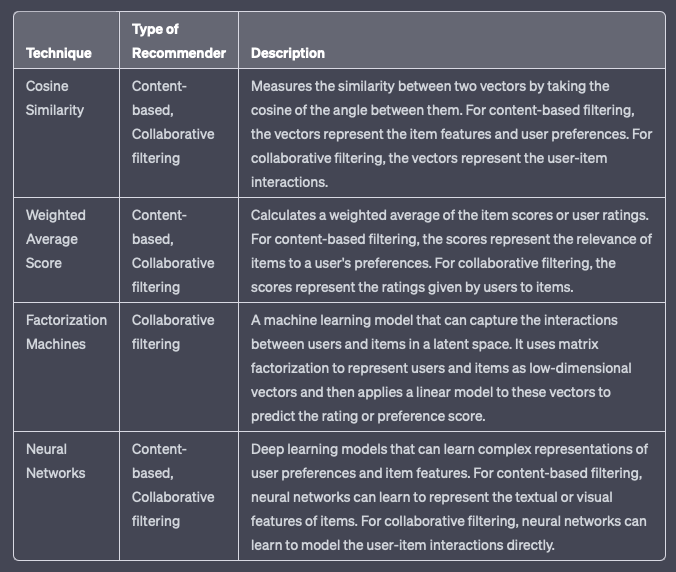
Candidate Ranking
- The candidate ranking step, formally known as the Learning to Rank (LTR) problem, involves selecting the most relevant items from the pool of candidate items to present to the user.
- Ranking can be done over multiple stages (which is typical in industry use-cases that rely on recommendations for monetization and success of their product). In the simplest dual-stage scenario, the typo stages may be called coarse and fine ranking. The various ranking options available are LTR methods, multi-armed bandits, wide-and-deep networks, etc.
- The first stage could involve LTR methods since they’re much easier to compute (and thus computation efficient) compared to other methods. Larger deep neural networks-based architectures are typically reserved for later stages, but that trend is slowly changing as compute efficiency is improving, thanks to innovation in ML acceleration.
- As a case study, let’s look at Instagram’s filtering approach for their “Explore” grid. In the candidate generation stage, account embeddings are used to identify accounts similar to those the user has previously interacted with. From these account candidates, they sample 500 media candidates (e.g., photos, stories).
- Then, these media candidates go through a three-pass ranking process which uses a combination of techniques to shrink neural network models:
- First pass: A distilled model mimics the later stages with minimal features to return the top 150 ranked candidates.
- Second pass: A lightweight neural network uses the full set of dense features and returns the top 50 ranked candidates.
- Final pass: A deep neural network uses the full set of dense and sparse features to return the top 25 ranked candidates (for the first page of the Explore grid).
- Instagram’s “funnel” architecture for multi-stage ranking is shown in the figure below (source).
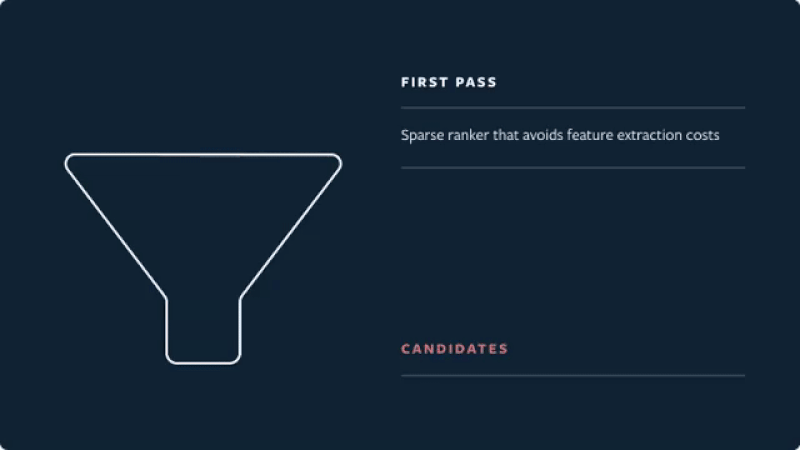
Learning to Rank (LTR)
- LTR methods aim to predict the probability that a user will interact with an item, given their previous interactions and other contextual information. LTR methods can be classified into three categories: (i) point-wise, (ii) pair-wise, and (iii) list-wise methods.
- The tables below give an overview of each technique and which method and recommender it is viable for.
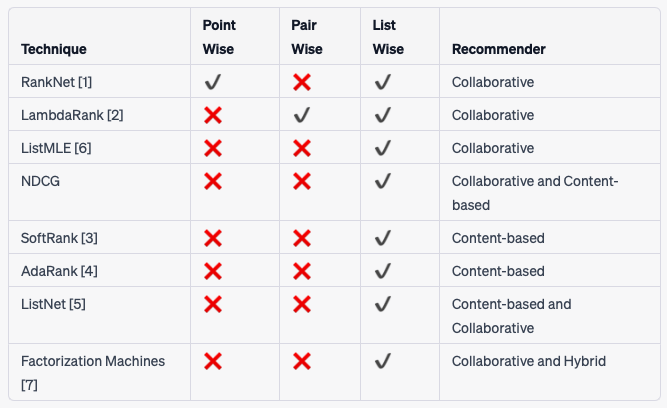
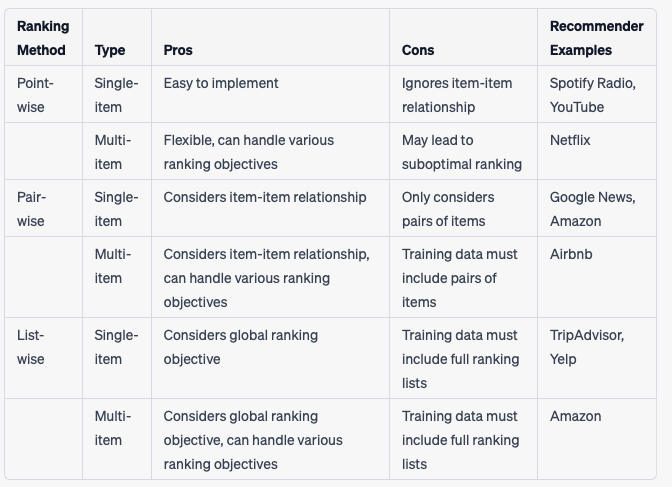
Point-wise methods
- Point-wise methods evaluate items independently, ignoring the rank order of the other items. A simple point-wise method is to use a linear model, such as logistic regression, to score each item based on a set of features.
- Point-wise approaches are a common method in learning-to-rank problems, where the focus is on predicting the relevance of a single document for a given query. In this approach, a classifier or regressor is trained to predict how relevant a document is for the query. The final ranking is obtained by sorting the list of results based on their individual document scores. Unlike other approaches, the score assigned to each document is independent of the scores assigned to the other documents in the result list for the same query.
- Point-wise approaches are relatively simple to implement and a wide range of regression and classification algorithms can be used in this approach. These algorithms take features of the document and query as input and predict a relevance score or class label. However, pointwise approaches do not take into account the interaction between documents and how they may compete with each other for the same query. As a result, they may not capture the relative importance of documents in the result list.
- Despite these limitations, point-wise approaches can be effective for certain types of ranking problems, especially when the relevance of each document is independent of other documents in the result list. For instance, pointwise approaches can be useful for ranking news articles or product recommendations, where each article or product has a unique relevance score.
Gradient Boosted Trees (GBT)
- Gradient Boosted Trees (GBT) is a pointwise ranking algorithm that is commonly used for recommender systems. GBT is an ensemble method that combines multiple decision trees to make predictions. It is based on gradient descent optimization and aims to optimize a ranking metric such as NDCG.
- GBT works by iteratively training decision trees on the negative gradients of the loss function. The negative gradients represent the direction in which the loss function decreases the most. The decision trees are trained to predict the negative gradients, and the predictions are added to the current model predictions to update the ranking scores.
- The process of iteratively training decision trees and adding the predictions to the current model predictions is repeated until a stopping criterion is met. The resulting ranking model assigns scores to each item, which can be used to rank the items for a given user.
Pair-wise methods
- Pair-wise methods compare items in pairs, and the goal is to learn a function that assigns a higher score to the preferred item in each pair.
- Pairwise approaches in learning to rank focus on pairs of documents in the loss function. Given a pair of documents, the goal is to determine the optimal order for that pair and compare it to the ground truth. The objective for the ranker is to minimize the number of inversions in ranking, which occur when the pair of results are in the wrong order compared to the ground truth.
- Pairwise approaches are preferred over pointwise approaches in practice because predicting the relative order of documents is more in line with the nature of ranking than predicting a class label or relevance score. Many of the most popular Learning to Rank algorithms, such as RankNet, LambdaRank, and LambdaMART, are based on pairwise approaches.
LambdaRank
- LambdaRank is a pairwise ranking algorithm that is commonly used for information retrieval and recommender systems. It is based on gradient descent optimization and aims to optimize a ranking metric such as NDCG.
- The basic idea behind LambdaRank is to use a gradient-based approach to adjust the pairwise preference function. The pairwise preference function is defined as the difference in relevance scores between two items for a given user. The goal is to learn a ranking model that assigns higher scores to more relevant items.
- LambdaRank uses gradient descent to iteratively update the model parameters. The gradient is calculated using the derivative of the ranking metric with respect to the model parameters. The gradient is then used to update the model parameters in the direction of maximum improvement in the ranking metric.
List-wise methods
- List-wise methods treat the ranked list of items as a whole and optimize a scoring function that directly maps from the item set to a ranking score.
- Listwise approaches in Learning to Rank directly examine the entire list of documents and attempt to determine the optimal ordering for it. There are two main sub-techniques for implementing listwise Learning to Rank.
- The first sub-technique is the direct optimization of information retrieval (IR) measures, such as Normalized Discounted Cumulative Gain (NDCG), which is a commonly used measure of ranking quality. This technique is used by algorithms such as SoftRank and AdaRank.
- The second sub-technique is minimizing a loss function that is defined based on an understanding of the unique properties of the ranking being targeted. For example, ListNet and ListMLE are algorithms that use this technique.
- While pointwise and pairwise approaches are simpler, listwise approaches can be more complicated. However, they are also considered more effective because they take the entire list into account, as opposed to just a single document or pair of documents.
Evaluation Metric: Normalized Discounted Cumulative Gain (NDCG)
- NDCG (Normalized Discounted Cumulative Gain) is a listwise ranking metric used to evaluate the quality of a recommender system’s ranked list of recommendations.
- In addition, other metrics such as Mean Reciprocal Rank (MRR), Average Reciprocal Hit Rate (ARHR), Mean Average Precision at \(k\) (mAP@\(k\)), and Mean Average Recall at \(k\) (mAR@\(k\)) are also used.
- For a detailed discourse on recommender systems’ metrics, please refer to our Evaluation, Metrics, and Loss primer.
LTR Algorithms: A Summary
- LTR algorithms are machine learning techniques used in information retrieval and recommender systems to rank items or documents based on user preferences or relevance to a given query. Here are a few commonly used LTR algorithms:
- Pointwise Methods: Pointwise methods treat ranking as a regression or classification problem by assigning a score or label to each item independently. Some popular pointwise methods include:
- Linear Regression: Fits a linear model to predict item scores based on features.
- Support Vector Machines (SVM): Maps features to a higher-dimensional space to find a hyperplane that separates relevant and irrelevant items.
- Logistic Regression: Applies logistic function to model the probability of an item being relevant.
- Pairwise Methods: Pairwise methods consider pairs of items and learn to compare their relative ranks. The algorithm is trained to rank one item higher than another when it is more relevant or preferred by users. Examples of pairwise methods include:
- RankNet: Utilizes a neural network to learn the ranking function by comparing pairs of items.
- RankBoost: Adapts boosting algorithms to learn a ranking function that minimizes pairwise misranking errors.
- RankSVM: Extends SVM to learn a ranking function by optimizing pairwise ranking constraints.
- Listwise Methods: Listwise methods aim to directly optimize the ranking of a list or a set of items. These methods consider the entire list as a single entity and learn a ranking function to directly optimize the listwise ranking metric. Notable listwise methods include:
- ListNet: Utilizes a neural network to directly learn the ranking probability distribution over lists of items.
- LambdaRank: Uses gradient boosting to optimize a listwise ranking objective, incorporating information about pairwise preferences.
- ListMLE: Maximum Likelihood Estimation approach that models the probability of the entire ranked list.
Position/Selection Bias in Scoring
- Items that appear lower on the screen are less likely to be clicked than items appearing higher on the screen. However, when scoring videos, the system usually doesn’t know where on the screen a link to that video will ultimately appear.
- Querying the model with all possible positions is too expensive. Even if querying multiple positions were feasible, the system still might not find a consistent ranking across multiple ranking scores as can be seen in the image below (source)

- For a more detailed analysis on positional bias, please refer the position/selection bias primer.
Putting it all together
Production model
- As an example, a production model (for say, CTR prediction for an ad on a page) with a 4 layer multi-layer perceptron (MLP) with dimensions of 512, 128, 64, and 16 with ReLU activation function and BatchNorm applied to all layers is as shown below.

- This model is fed with the concatenation of textual, numerical, and categorical features.
- The textual features are converted to dense representation by:
- Tokenizing the input.
- Generating hash value for each token.
- Using the hash value to lookup corresponding embedding from the embedding table, and
- Performing mean-pool operation on the retrieved embedding vectors to generate a single dense representation for the text (e.g., the title of the product page).
- The categorical features (e.g., page category) are converted to a one-hot encoding and the corresponding embedding is retrieved from a randomly initialized embedding table.
- All the aforementioned features are concatenated and added as input to the MLP model with the click/non-click binary label and trained with binary cross entropy loss function.
Challenges in Scaling Up Models on Noisy Datasets
- Simply scaling up the model size without any other changes to the fine-tuning methodology can result in a drop in performance. Different techniques should be explored to mitigate this drop in performance and improve the training of larger models for noisy datasets (e.g., CTR data).
- In practice, reverse distillation (where we transfer knowledge from the smaller models to larger models) is an important technique to improve the performance and training stability of larger models, especially with inherently noisy datasets like CTR. In addition to this, projecting the semantic embeddings down to a lower dimension to allow better interaction with behavioral features and warm-starting the models with pre-trained data can also help improve the performance of larger models.
YouTube ranking
- The YouTube ranking architecture (source) is depicted in the architecture below.
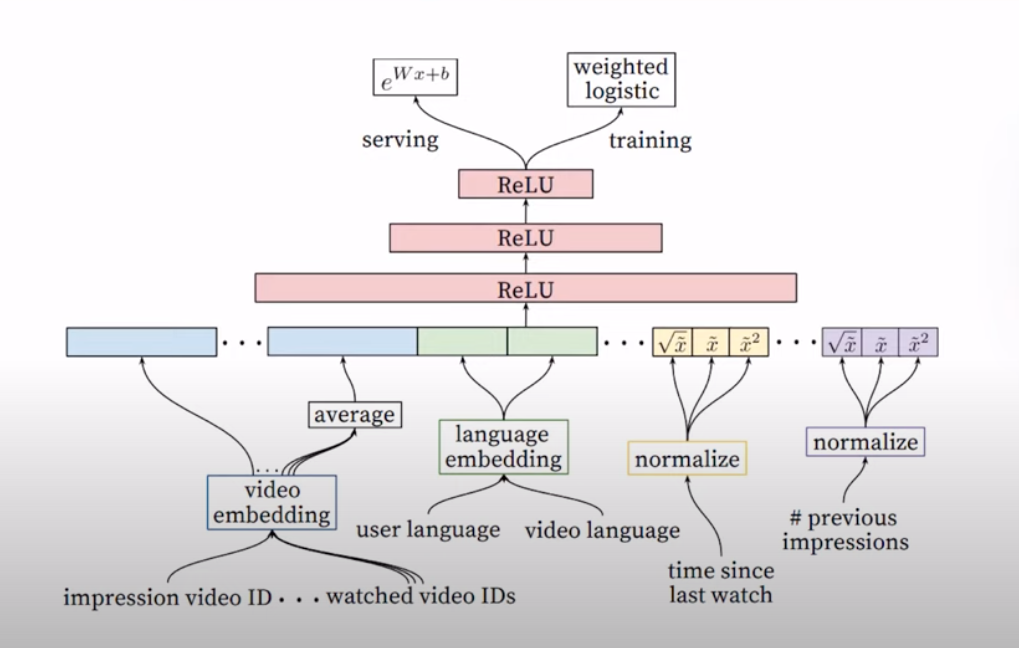
- The ranking stage operates on hundreds of videos and outputs about a dozen.
- The input to the architecture is a pair of user and video embeddings.
- During training, it leverages a weighted logistic regression model which will give you a score between 0 or 1 and that will be the ranking.
- The user and video features that go into the model are previous impressions, time since last watch, user and video language.
- It passes through feed forward network with ReLU activation as well.
- Note, this architecture takes in 1 video and 1 user at one time and iterates this over all candidates but you could have multiple servers running this in parallel.
References